
eLaw publishes article in Computer Law & Security Review
In healthcare, gender and sex considerations are crucial because they affect individuals' health and disease differences. Yet, most algorithms deployed in the healthcare context lack close consideration of these aspects and do not account for bias detection. In their latest paper, Eduard Fosch-Villaronga, Hadassah Drukarch, Pranav Khanna, Tessa Verhoef and Bart Custers stress how missing these dimensions is a huge point of concern, as neglecting these aspects will inevitably produce far from optimal results and generate errors that may lead to misdiagnosis and potential discrimination.
AI has gained immense popularity within the healthcare domain, where it promises to find and use complex underlying relationships between the way humans work and how to care for them to improve care, discover new treatments for a wide variety of diseases, and advance scientific hypotheses even if we as humans do not necessarily understand those underlying relationships.
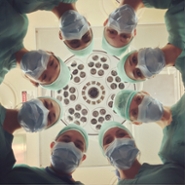
Yet while these advances may entail incredible progress for medicine and healthcare delivery soon, more research is needed for these systems to perform well in the wild, especially in the area of diversity and inclusion. In particular in the healthcare domain, AI can be considered a 'double-edged sword'. While, here, AI is used to predict, address or support a health-related decision, errors may compromise safety and allow for misdiagnosis, a massive problem that, paradoxically, AI is trying to solve.
Eduard Fosch-Villaronga, Hadassah Drukarch, Pranav Khanna, and Bart Custers from eLaw - Center for Law and Digital Technologies in collaboration with Tessa Verhoef from the Creative Intelligence Lab (CIL) and LIACS at Leiden University have just published an article on Accounting for diversity in AI for Medicine in the prestigious journal Computer Law and Security Review that explores how algorithm-based systems in healthcare related applications may reinforce gender biases and have inadvertent discriminatory, safety, and privacy-related impacts on marginalised communities. By promoting the account for privacy, safety, diversity, and inclusion in algorithmic developments with health-related outcomes, the authors ultimately aim to inform the AI global governance landscape and practice on the importance of integrating gender and sex considerations in the development of algorithms to avoid exacerbating existing or new prejudices.
This article comes as a result of the collaboration established by the Gendering Algorithms initiative at Leiden University, a project aiming to explore the functioning, effects and governance policies of AI-based gender classification systems. Gendering algorithms is chaired by Eduard Fosch-Villaronga and Tessa Verhoef and was funded by the Global Transformations and Governance Challenges program at Leiden University.